Six Ways Machine Learning Will Transform the Biopharmaceutical Lifecycle
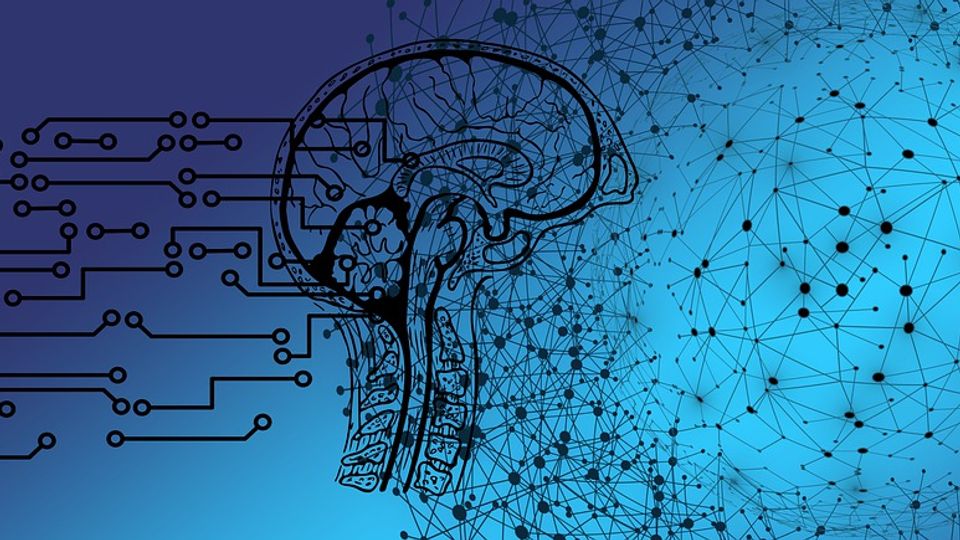
Generative artificial intelligence (AI) tools, such as GPT-4 for text and Midjourney for images, have astonished the public over the last year. Many people were simply unaware of the potential for artificial intelligence – and machine learning (ML) in particular – to reshape industries.
In the biopharmaceutical industry, machine learning is already changing drug development and production. Over the next decade, it will accelerate the biopharmaceutical lifecycle by making processes more efficient and democratizing insights. Attaining these eagerly awaited advances, though, will involve a paradigm shift in what it means to manage data well.
Here are four ways that AI and ML are accelerating the biopharmaceutical lifecycle and two big ways that companies are starting to think about their data differently.
Accelerating drug discovery
It is misleading to say that computers could ever be smart in the same way as humans, since brains and machines process information very differently. But in targeted ways, artificial intelligence is already outpacing human ability – particularly when it comes to spotting patterns in huge data sets.
For example, some AI models can summarize and analyze scientific texts better than traditional natural language processing systems and orders of magnitude faster than humans. These models could be used, for example, to find potential new drug–disease associations. Other models, focused on chemical structure, can automatically optimize molecule design, improving properties such as binding affinity or toxicity.
Improving experimental design
Once new drugs are developed, figuring out how to produce them in the most efficient way is also a time-consuming challenge.
When using a bioreactor to create a therapeutic protein from cell culture, variables to consider include raw ingredients, temperature and pressure. Adjusting the levels of each variable to optimize yield usually involves lots of trial and error. Process development scientists painstakingly tweak variables one by one – a warmer temperature makes cells grow faster, but if too hot then it stalls, for example.
With hundreds of parameters and multiple cell cultures to consider, this work can be labor-intensive, slow and inefficient. Now, using advanced analytics tools, multivariate analysis can quickly narrow down the handful of variables likely to matter most and identify how they relate to each other – temperature, for instance, can affect pH. Then, machine learning techniques can generate experimental designs and recommend exactly which experiments to run – and in which order – to figure out the best values for each.
Streamlining production processes
Experimental design modeling is an important part of the early stages of production. Throughout the optimization and definition of the production process, it is critical to capture a full picture of the data generated in development. Only by recording how the process and the product interact – and removing the bias towards “success” data – can we begin to see the full benefits of AI and other advanced analytics for process optimization.
Drugs must be produced consistently to ensure purity and potency, and when scaling up from small bioreactors to million-liter tanks, mistakes that compromise these factors are much more wasteful. This is where AI can help to save time and money in drug manufacturing.
Digital twins of bioreactors that make use of machine learning can be created, even before starting real runs, to optimize the process. As the scale changes, the conditions inside of the bioreactor change, but performing virtual testing can anticipate these variations, leading to significant time savings. However, technicians still need to spot outliers right away. That means knowing from the start which levels are in scope. To identify optimal levels, data scientists can average dozens of runs in a small-scale bioreactor, create models using those runs and then test the models to identify exact targets for each variable.
Democratizing insights
By analyzing huge data sets and predicting patterns, AI and ML are already speeding up early research and development, drug production design and the manufacturing process at scale. They are also speeding up the biopharmaceutical lifecycle in another way: by making insights more accessible.
In the past, people at the bench had to rely on data science teams to both acquire the data and interpret it. Now, these data science teams help to create tools that empower users to extract insightful information, derived from the original data, and create dashboards for an even easier interpretation.
In the lab and on the factory floor, machine learning can simplify dashboards by identifying the most important variables and how they interact. Instead of monitoring 20 parameters, technicians can monitor one graph showing deviation from the correct path – saving time and improving outcomes.
For managers, machine learning techniques can provide more visibility across silos. Many teams store research papers or notes in the cloud, and large language models like GPT-4 can summarize these documents or parse them for keywords. Managers can then spot teams that are working on similar problems and better support information sharing.
Redefining which data matter
All these advances, however, require new ways of thinking about data; the saying "the process is the product" has never been truer. For one thing, machine learning models are only as smart as the data they are trained on.
Take a model that will automatically analyze cell purity using flow cytometry data in your drug development process. For the model to categorize samples, humans need to tag an initial batch. For the model to be accurate, that first batch needs to be balanced; it should contain roughly as many high purity cell samples as low purity ones. This is true when there are multiple variables at play too. For instance, a model designed to spot outliers in high-throughput screenings will need lots of examples of diverse kinds of outliers.
A shift in thinking is required to produce good training data. In the past, the norm was to hit on a process that worked and then scale it up without deeply understanding why the process worked. Many teams focused on tracking success, and they often discarded "failure" data. Similarly, researchers often only published successful experiments, and did not bother to send null results through for peer review. Now, outliers and mistakes are valuable; those data are needed to create accurate models. Teams need a plan for collecting balanced data sets—or for generating failure data synthetically.
Changing how data are stored
In addition to changing which data matter, machine learning is also changing how data must be stored. For many companies, this is often the bigger challenge.
Data scientists can work with structured and unstructured data, images, documents, readings from IoT devices and more. But to use those data sets, they need to be able to find them. To be accessible, data should be reachable using a system sometimes known as a data backbone. It should also come with clear metadata and context, so data scientists know what they are looking at.
Data that are organized enough for AI to interpret also offer further benefits. Organized data save data scientists’ time so they can focus on higher-level projects. It can also enable plug-and-play machine learning models that end users can apply independently, democratizing insights further. Finally, it can reveal hidden relationships across silos and help upstream and downstream teams to transfer information more easily.
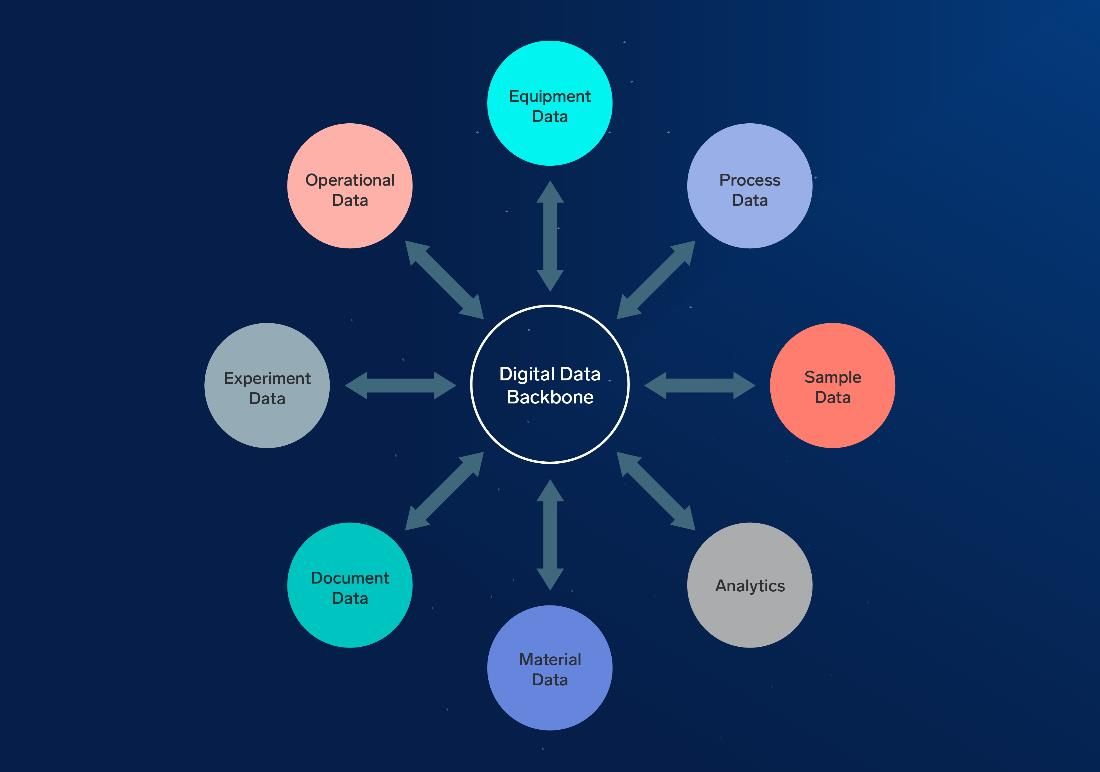
Figure 1: Data types to capture the meaningful experimental context needed to unlock the power of AI/ML models. Courtesy of IDBS.
Implementing a new strategy for data management is a big undertaking. But along the way, companies will likely stumble on surprising efficiencies and uncover things they would not have uncovered on their own. Given how much AI and ML can speed time to market, the investment is worth it.
About the author:
Daniel Tabas works as a senior data scientist in the Data Science & Analytics group at IDBS. He is a computer scientist with a PhD in Bioinformatics, specialized in data science, analytics and artificial intelligence, and with wide experience in the biomedical/biopharma domains. After obtaining his BSc in Computer Science in the Complutense University of Madrid, he joined the Spanish National Center for Biotechnology, where he worked in a core facility group while he completed his PhD in Bioinformatics. Later, he worked in PerkinElmer as a principal AI engineer.