Exploring the Latest Advances in Transcriptomics
Advancements in transcriptomic technology have enabled many new discoveries in recent years.
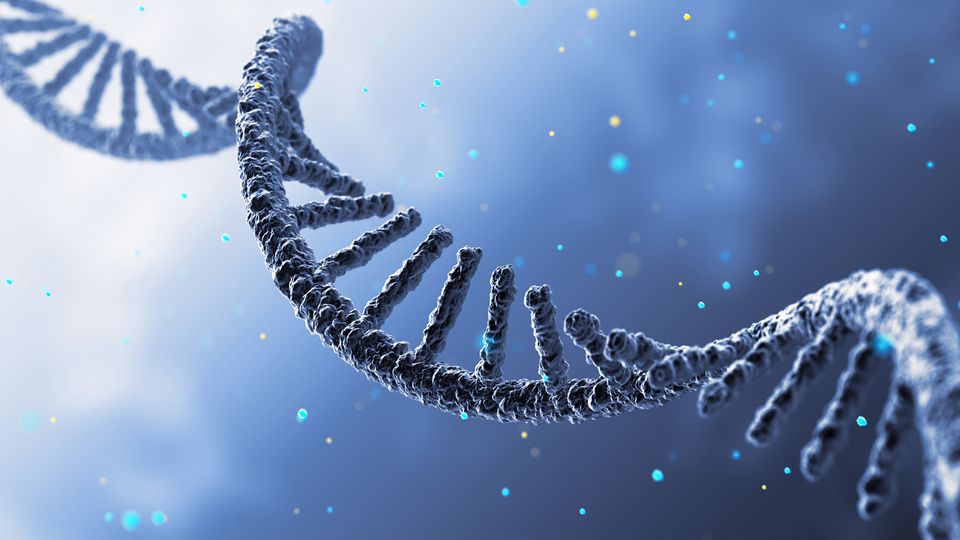
Complete the form below to unlock access to ALL audio articles.
Since the idea of genetic regulation was first postulated back in the 1950s, our scientific understanding of the transcriptome has deepened greatly.1 Looking into the transcriptome of cells and tissues has helped scientists to understand the biological processes that drive both health and disease; however, the complex and occasionally mysterious nature of genetic regulation continues to raise questions among scientists today. Not least, the diversity, heterogeneity and environmental susceptibility of complex transcriptomes leave many researchers challenged by the limitations of technology.2,3
The central dogma of gene expression states that information flows in a single direction, from the inherited genetic code (DNA) to functional proteins via ribonucleic acid (RNA) intermediaries.4,5 While it is now known that many gene expression systems are far more complicated than this, the concept still forms the basis of many differential expression studies. In fact, multiple RNA construct types – such as messenger RNA (mRNA), transfer RNA (tRNA) and microRNA – all work together as a cohesive system, to modulate the transcription and translation of genetic information.
Through a detailed understanding of gene expression, scientists can infer vast quantities of information about the role of gene regulation in health and disease. Mostly, this has been done by examining differential expression patterns in RNA sequencing (RNAseq) studies. The detection of RNA molecules and the analysis of their relative abundance have provided scientists with unparalleled insights into the genes and gene sets being actively transcribed within a cell or tissue at a given time. This method has come to define the experimental approach now known as widely as “transcriptomics”.
This article explores the latest technologies currently in use for transcriptomic research. It also highlights the latest advancements in transcriptomics technology, focusing on single-cell, spatiotemporal and the “dark-transcriptome”.
What is transcriptomics?
Transcriptomics is the study of the total set coding and/or non-coding RNA constructs that are present within a cell or cell population.6 It can also be used to identify differential expression patterns in cells of different types, tissues, differentiation, or disease states.
mRNA was once considered to be the most important ribonucleic acid present in eukaryotic cells due to its ability to translate genomic information into functional proteins.7 However, scientists now acknowledge the critical importance of other RNA specifies, outlined in Table 1. In recent considerations regarding the importance of other RNA transcript types has driven the development of new transcriptomic technologies.8
Table 1: Types of RNA that make up the transcriptome. Credit: Technology Networks.
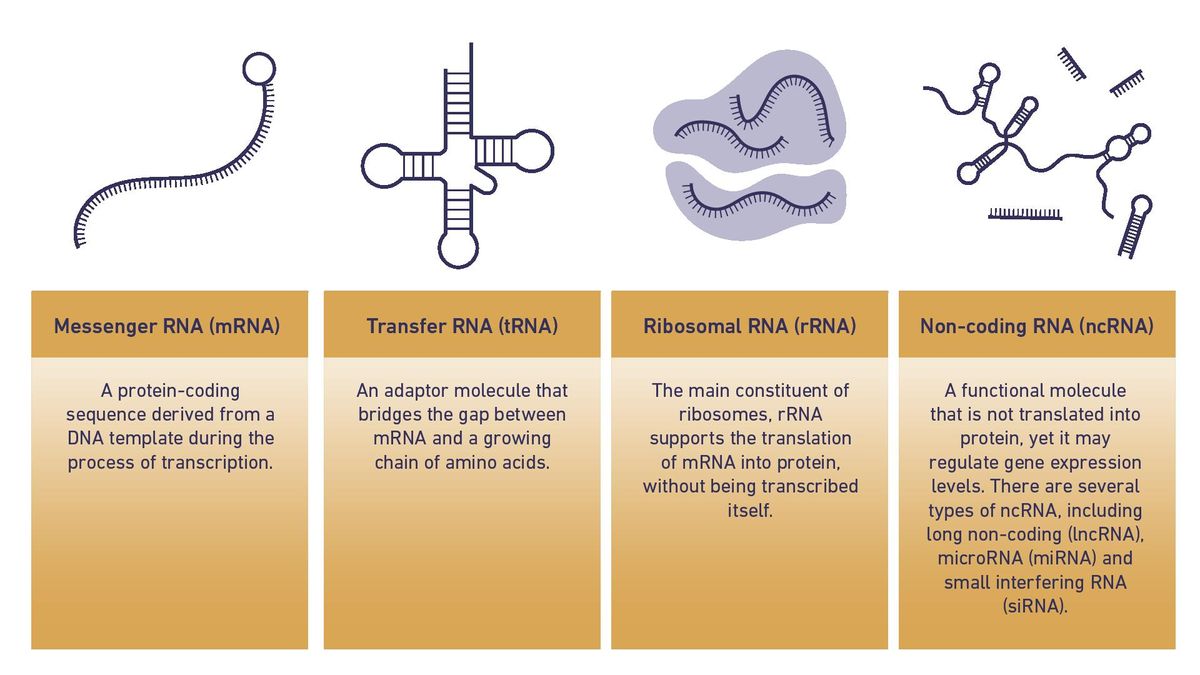
Transcriptomics technologies and their current applications
Several different transcriptomic technologies exist, with each offering unique advantages and challenges for research. While technologies may vary in their methodological approach, all rely on RNAseq to identify and quantify gene transcripts.9 Modern RNAseq achieves this through a massively parallel approach, enabling the detection of multiple transcript fragments simultaneously.
RNAseq technologies can be broadly categorized into either short-read or long-read approaches. Short-read sequencing offers greater affordability and higher confidence DNA base calling.10 However, it also relies on lengthy workflows and holds a preferential bias towards certain fragment types. Long-read approaches enable the continuous sequencing of larger, intact RNA transcripts, enhancing the discovery power of previously unknown or non-coding RNA species.11
Advancements in transcriptomic technologies
In recent years, advancements in transcriptomic technologies have propelled the field beyond the fundamental aspects of gene expression research.12 Notably, RNAseq workflows have become faster, higher-throughput and more accurate due to the rise of genomic surveillance in public health applications.13,14 Today, RNAseq is used to facilitate the discovery of novel transcripts and splice variants, for mutation detection in both single-cell prokaryotic and eukaryotic sample types, and in personalized medicine.15,16,17,18
Dr. Alexander Stark, senior group leader at the Research Institute of Molecular Pathology (IMP), Vienna commented on the advancements in transcriptomics technology that he has found particularly exciting: “To me, the ability to determine the transcription profiles of individual cells within complex tissues like the mammalian brain and others is very exciting. It allows the comprehensive identification of all cell types in the respective tissue and ultimately the entire human organism. It also allows detailed analyses of how cellular transcription and cell state get derailed in disease.”
Single-cell transcriptomics
In complex tissues gene transcription may vary with spatial context.19 Hence, single-cell RNA sequencing (scRNA-seq) has proven a revolutionary tool in providing greater resolution in transcriptomic studies.
Previously, bulk RNA sequencing provided an average gene expression profile across a mixture of cell or cell types masking crucial information about individual cells’ transcriptomes. Today, scRNA-seq allows researchers to look deeper into profile of individual cells to build a more comprehensive picture of how cells exist in relative context.20 scRNA-seq has proven particularly valuable in elucidating the heterogeneity of complex tissues and in revealing previously hidden cellular subtypes.21,22,23
Several technological advancements have been necessary to enable the development of scRNA-seq methods. Namely, cell isolation approaches such as fluorescent activated cell sorting (FACS) and microfluidic methods have been essential in preparing sample material for sequencing.24 In addition, the development of sequencing platforms to adopt droplet-based technologies has also facilitated the advancement of single-cell methods.25
Stark’s team uses a technology called single-cell sequencing assay for transposase-accessible chromatin (scATAC-seq) to determine the regulatory genomic elements in individual cells and cell types. Unlike RNA sequencing, which focuses on the transcribed genome, ATAC-seq provides researchers a genome-wide view of the accessible regions of chromatin.
“Very recently, in collaboration with the Eileen Furlong lab, we have used scATAC-seq data in combination with enhancer activity data to train deep-learning models that are able to predict enhancer activities directly from the DNA sequence,” explained Stark. For a cell to establish its distinct identity, form and function, the activation of genes via "enhancers" is necessary. By leveraging genomics and artificial intelligence (AI) tools, Stark and Furlong have been able to unravel this second "code of life".
Stark continues, “We have then used these models to design and build synthetic enhancers for five tissues in the fruit fly embryo.” This work was recently published in Nature, and Technology Networks recently interviewed Stark about the key study findings.
Spatial and spatiotemporal transcriptomics
To achieve even greater resolution, scRNA-seq methods have been coupled with other methodologies, such as image or capture-based technologies to yield spatial information about localized transcription within cells and organelles.20
“It's important to use the two different kinds of technologies in combination,” said Dr. Hongkui Zeng, director of the Allen Institute for Brain Science.
Since joining the institute, Zheng has spearheaded various initiatives to create and manage high-throughput pipelines, facilitating the production of extensive open-access datasets and tools to accelerate neuroscience discovery. Specifically, her team develops various platforms ‒ single-cell transcriptomics, brain-wide connectivity mapping, neuronal activity imaging, etc. ‒ to systematically characterize the transcriptomic, physiological, morphological and connectional features of diverse neuron types.
“The two main things we are looking for, are scalability, so larger areas can be efficiently profiled, and also depth of coverage, so more genes can be profiled, while still maintaining the high resolution, the single-cellular resolution. Those are critical issues we come across when we try to adopt a new spatial technology,” said Zeng.
By performing RNA sequencing, it is possible to obtain the expression profiles of all the genes, in an unbiased manner. She continues: “scRNA-seq data gives you the depth and quantitative profiling of all the genes in the genome.”
“Currently, spatial technologies are limited in their depth of coverage. Targeted approaches only allow us to look at hundreds of genes, a thousand at a stretch ‒ that's far from the entire transcriptome or genome,” noted Zheng. This is why researchers still currently rely on RNA sequencing, to obtain whole transcriptome-wide data.
“Of course, someday, if the spatial method provides enough sensitivity that allows you to profile more genes ‒ 5000 or 10,000 genes, for example ‒ maybe you wouldn’t need the sequencing. You’d just use the spatial method,” Zheng postulated.
Image-based approaches utilize either FISH or in-situ sequencing (ISS) to detect individual RNA molecules within intact cells or tissues. FISH-based methods include cell fixation and in-situ hybridization steps prior to standard scRNA-seq. ISS-based methods incorporate a wholly different approach, using fluorescent probes to determine transcript sequences within intact, fixed cells.
Alongside the importance of spatial context, the temporal expression of RNA transcripts is now also recognized as a crucial aspect of gene regulation.26 Hence, spatiotemporal approaches are now used to explore how transcriptomic profiles evolve within single cells over time. Such methods utilize fluorescent labeling technology and complex cell culture approaches to visualize fixed transcripts in live single-cell populations. As credence is increasingly given to the importance of non-coding RNA species, spatiotemporal transcriptomics has proven insightful into research regarding lncRNAs, which exhibit developmental stage specificity and high turnover rates.27,28,29
Long-read sequencing is helping to unravel the dark transcriptome
Despite recent advances in transcriptomic technology, almost all methods exhibit some annotation biases toward protein coding genes.30 While some biases occur during the sequencing process itself, others are introduced during bioinformatics steps and data processing.31 Hence, knowledge gaps remain in the annotation of complex transcriptomes, despite a scientific awareness that such biases exist. Transcripts which arise from poorly annotated gene regions have come to be classified as the “dark transcriptome”.32
Long-read sequencing is being increasingly applied to help unravel the dark transcriptome.30 Long-read technologies offer scientists to opportunity to overcome sequencing biases toward high-abundance transcripts and repeat sequences, by eliminating the fragment biases of short-read amplicon-based methods.
In order to address the effects of historic sequencing biases, efforts have also been made to reassess existing transcriptomic data, applying new methods to old datasets. Hence, the discovery power held within the dark transcriptome is intertwined with the concurrent advancement in computational technology and software pipelines.
Conclusion
Advancements in transcriptomic technology have enabled many new discoveries in recent years, from the genomic surveillance of single-cell pathogens to the high-resolution analysis of complex eukaryotic tissues. Scientists’ ability to examine gene expression at single-cell resolution, study spatial transcriptomics, and visualize RNA molecules at the single-molecule level has transformed our understanding of biology; however, mysteries still remain in poorly annotated regions of the dark transcriptome. While long-read technologies and advanced gene annotation methods are helping researchers advance their understanding of the transcriptome, there will always remain new discoveries to be made as mutations occur and as lifeforms evolve.
References
1. Morange M. François Jacob (1920-2013). Nature. 2013;497(7450):440. doi: 10.1038/497440a
2. Choe K, Pak U, Pang Y, Hao W, Yang X. Advances and challenges in spatial transcriptomics for developmental biology. Biomolecules. 2023;13(1):156. doi: 10.3390/biom13010156
3. Atta L, Fan J. Computational challenges and opportunities in spatially resolved transcriptomic data analysis. Nat Commun. 2021;12(1):5283. doi: 10.1038/s41467-021-25557-9
4. Crick F. Central dogma of molecular biology. Nature. 1970;227(5258):561-563. doi: 10.1038/227561a0
5. , The central dogma revisited: insights from protein synthesis, CRISPR, and beyond. Wiley Interdiscip. Rev. RNA. 2022;13(5),e1718.doi: , . 10.1002/wrna.1718
6. Lowe R, Shirley N, Bleackley M, Dolan S, Shafee T. Transcriptomics technologies. PLoS Comput Biol. 2017;13(5):e1005457. doi: 10.1371/journal.pcbi.1005457
7. Diamantopoulos MA, Tsiakanikas P, Scorilas A. Non-coding RNAs: the riddle of the transcriptome and their perspectives in cancer. Ann Transl Med. 2018;6(12):241. doi: 10.21037/atm.2018.06.10
8. Sun, YM, Chen, YQ. Principles and innovative technologies for decrypting noncoding RNAs: from discovery and functional prediction to clinical application. J Hematol Oncol. 2020;13:109. doi: 10.1186/s13045-020-00945-8
9. Wang Z, Gerstein M, Snyder M. RNA-seq: a revolutionary tool for transcriptomics. Nat Rev Genet. 2009;10(1):57-63. doi: 10.1038/nrg2484
10. Adewale BA. Will long-read sequencing technologies replace short-read sequencing technologies in the next 10 years? Afr J Lab Med. 2020;9(1):1340. Doi: 10.4102/ajlm.v9i1.1340
11. Begum G, Albanna A, Bankapur A, et al. Long-read sequencing improves the detection of structural variations impacting complex non-coding elements of the genome. Int J Mol Sci. 2021;22(4):2060. doi: 10.3390/ijms22042060
12. Conte MI, Fuentes-Trillo A, Domínguez Conde C. Opportunities and tradeoffs in single-cell transcriptomic technologies. Trends Genet. doi: 10.1016/j.tig.2023.10.003
13. Novelli G, Biancolella M, Mehrian-Shai R, et al. COVID-19 one year into the pandemic: from genetics and genomics to therapy, vaccination, and policy. Hum Genomics. 2021;15(1):27. doi: 10.1186/s40246-021-00326-3
14. The Lancet Editorial. Genomic sequencing in pandemics. Lancet. 2021;397(10273):445. doi: 10.1016/S0140-6736(21)00257-9
15. Van de Sande B, Lee JS, Mutasa-Gottgens E, et al. Applications of single-cell RNA sequencing in drug discovery and development. Nat Rev Drug Discov. 2023;22(6):496-520. doi: 10.1038/s41573-023-00688-4
16. Lang J, Cho WC, Huang T, Wu T, Xu J. Applications of RNA-seq in cancer and tumor research. Front Genet. 2023;14:1331576. doi: 10.3389/fgene.2023.1331576
17. Stockdale SR, Blanchard AM, Nayak A, et al. RNA-seq of untreated wastewater to assess COVID-19 and emerging and endemic viruses for public health surveillance. Lancet Reg Health Southeast Asia. 2023;14:100205. doi: 10.1016/j.lansea.2023.100205
18. Kim J, Park J. Single-cell transcriptomics: a novel precision medicine technique in nephrology. Korean J Intern Med. 2021;36(3):479-490. doi: 10.3904/kjim.2020.415
19. Lebrigand K, Bergenstråhle J, Thrane K, et al. The spatial landscape of gene expression isoforms in tissue sections. Nucleic Acids Res. 2023;51(8):e47. doi: 10.1093/nar/gkad169
20. Lee J, Yoo M, Choi J. Recent advances in spatially resolved transcriptomics: challenges and opportunities. BMB Rep. 2022;55(3):113-124. doi: 10.5483/BMBRep.2022.55.3.014
21. Kim SH, Cho SY. Single-cell transcriptomics to understand the cellular heterogeneity in toxicology. Mol. Cell. Toxicol. 2023;19:223-228 (2023). doi: 10.1007/s13273-022-00304-3
22. Saviano A, Henderson NC, Baumert TF. Single-cell genomics and spatial transcriptomics: Discovery of novel cell states and cellular interactions in liver physiology and disease biology. J Hepatol. 2020;73(5):1219-1230. doi: 10.1016/j.jhep.2020.06.004
23. Brown CC, Gudjonson H, Pritykin Y, et al. Transcriptional basis of mouse and human dendritic cell heterogeneity. Cell. 2019;179(4):846-863.e24. doi: 10.1016/j.cell.2019.09.035
24. Liu Y, Fan Z, Qiao L, Liu B, Advances in microfluidic strategies for single-cell research, TrAC, Trends Anal. Chem. 2020;157:116822. doi: 10.1016/j.trac.2022.116822
25. Muskovic, W, Powell, JE. DropletQC: improved identification of empty droplets and damaged cells in single-cell RNA-seq data. Genome Biol. 2021;22:329. doi: 10.1186/s13059-021-02547-0
26. Ren J, Zhou H, Zeng H, et al. Spatiotemporally resolved transcriptomics reveals the subcellular RNA kinetic landscape. Nat Methods. 2023;20(5):695-705. doi: 10.1038/s41592-023-01829-8
27. Mattick JS, Amaral PP, Carninci P, et al. Long non-coding RNAs: definitions, functions, challenges and recommendations. Nat Rev Mol Cell Biol. 2023;24(6):430-447. doi: 10.1038/s41580-022-00566-8
28. Pang J, Zhang X, Ma X, Zhao J. Spatio-temporal transcriptional dynamics of maize long non-coding RNAs responsive to drought stress. Genes (Basel). 2019;10(2):138. doi: 10.3390/genes10020138
29. Liau WS, Samaddar S, Banerjee S, Bredy TW. On the functional relevance of spatiotemporally-specific patterns of experience-dependent long noncoding RNA expression in the brain. RNA Biol. 2021;18(7):1025-1036. doi: 10.1080/15476286.2020.1868165
30. Kuo RI, Cheng Y, Zhang R, et al. Illuminating the dark side of the human transcriptome with long read transcript sequencing. BMC Genom. 2020;21(1):751. doi: 10.1186/s12864-020-07123-7
31. Finotello F, Lavezzo E, Bianco L, et al. Reducing bias in RNA sequencing data: a novel approach to compute counts. BMC Bioinform. 2014;15(Suppl 1):S7. doi: 10.1186/1471-2105-15-S1-S7
32. Li J, Singh U, Arendsee Z, Wurtele ES. Landscape of the dark transcriptome revealed through re-mining massive RNA-seq data. Front Genet. 2021;12:722981. doi: 10.3389/fgene.2021.722981